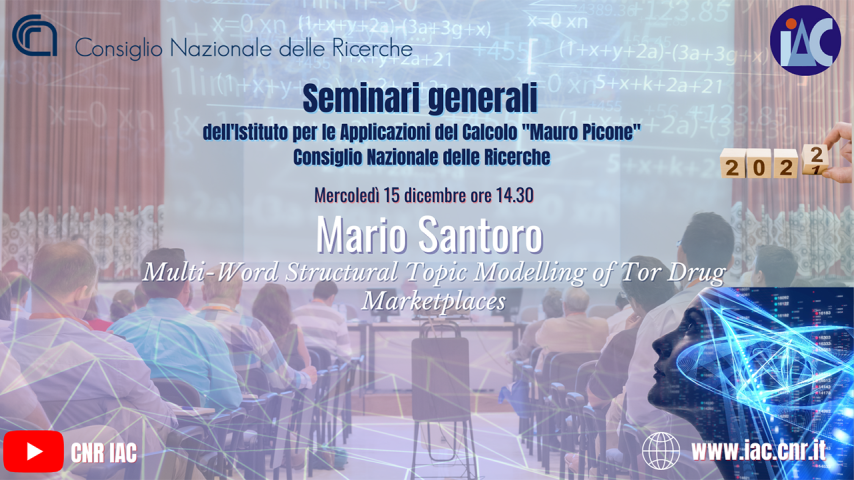
Topic Modelling (TM) is a widely adopted generative model used to infer the thematic organization of text corpora. When document-level covariate information is available, so-called Structural Topic Modelling (STM) is the state-of-the-art approach to embed this information in the topic mining algorithm. Usually, TM algorithms rely on unigrams as the basic text generation unit, whereas the quality and intelligibility of the identified topics would significantly benefit from the detection and usage of topical phrasemes. Following on from previous research, in this paper we propose the first iterative algorithm to extend STM with n-grams, and we test our solution on textual data collected from four well-known ToR drug marketplaces. Significantly, we employ a STM-guided n-gram selection process, so that topic-specific phrasemes can be identified regardless of their global relevance in the corpus. Our experiments show that enriching the dictionary with selected n-grams improves the usability of STM, allowing the discovery of key information hidden in an apparently “mono-thematic” dataset.