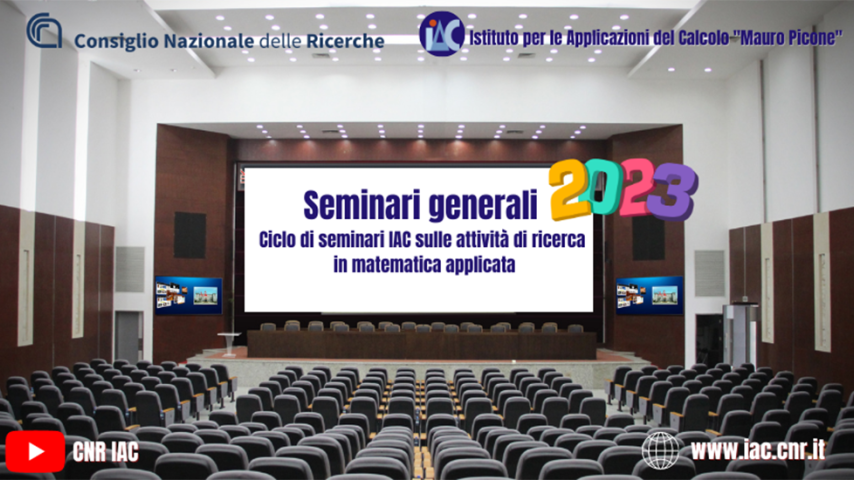
Questi brevi workshop sono dedicati alle attività di ricerca in matematica applicata.
I seminari generali IAC sono l'occasione per ricercatori dell'istituto e non di presentare le loro ricerche a un pubblico più vasto di quello del proprio settore di riferimento nella matematica applicata. Il linguaggio scelto non è, infatti, strettamente tecnico e si favorisce la fruizione a un pubblico più esteso, per stimolare collaborazioni interdisciplinari.
Tutti i seminari sono trasmessi in streaming sul canale Youtube dell'istituto @CNRIAC.
I seminari si tengono sempre alle ore 14:30.
Di seguito il calendario dei seminari in programma:
12 luglio - Irène Gijbels, Full Professor al Dipartimento di matematica della KU Leuven
Titolo: On hybrid hazard based regression models for censored data.
Abstract
In the analysis of time-to-event or survival data, the Cox proportional hazard model and the accelerated failure time model, are well-known models. In both models, the effect of covariates is standard of a parametric form. In this talk, we consider a hybrid hazard-based regression model that englobes both mentioned models, among others, and in addition, allows for more flexible (non-specified) effects of the covariates. The focus is on revealing the impact of the covariates, whereas the baseline hazard is modelled via a large class of two-piece asymmetric baseline distributions. We discuss estimation methods in various settings (parametric, semi-parametric -- partly linear, and non-parametric). We investigate the performance of the estimators, and illustrate the practical use of the developed methods in real data applications. This talk is based on joint work with Worku Biyadgie Ewnetu (UHasselt and KU Leuven) and Anneleen Verhasselt (UHasselt).
Elenco seminari passati
11 gennaio - Stefano Zaghi, ricercatore dell'IAC
Titolo "Adaptive Mesh Refinement CFD Simulations in the Exascale Era".
Abstract
A discussion concerning the reasons to develop a new Adaptive Mesh Refinement (AMR) CFD library, the challenges of leveraging modern exascale supercomputers' computational resources, and the author's current failures (with some, probably negligible, progress).
25 gennaio - Adriano Tiribocchi, ricercatore dell'IAC
Titolo "Modeling the collective properties of multi-core emulsions under confinement"
Abstract
Multi-core emulsions are a class of soft fluids assembled from cluster configurations of deformable oil-water double droplets (cores), often employed as building-blocks for the realisation of devices of interest in biotechnology, drug delivery and tissue engineering. We use lattice Boltzmann simulations to study the physics of multi-core emulsions flowing in microfluidic channels and report numerical evidence of a surprisingly rich variety of driven non-equilibrium states, whose formation is caused by a dipolar fluid vortex triggered by the sheared structure of the flow carrier within the microchannel. If time permits, we will also discuss the translocation dynamics of emulsion drops with multiple close-packed inner droplets flowing within constrictions. Under such conditions, these liquid architectures display a number of non-trivial features, such as permanent shape deformations and memory-like effects, of particular relevance for the design of soft porous materials.
8 febbraio - Luca Galantucci, ricercatore dell'IAC
Titolo "Dissipation anomaly in a turbulent quantum fluid"
Abstract
When the intensity of turbulence is increased (by increasing the Reynolds number, e.g. by reducing the viscosity of the fluid), the rate of the dissipation of kinetic energy decreases but does not tend asymptotically to zero: it levels off to a non-zero constant as smaller and smaller vortical flow structures are generated. This fundamental property, called the dissipation anomaly, is sometimes referred to as the zeroth law of turbulence. The question of what happens in the limit of vanishing viscosity (purely hypothetical in classical fluids) acquires a particular physical significance in the context of liquid helium, a quantum fluid which becomes effectively inviscid at low temperatures achievable in the laboratory. By performing numerical simulations and identifying the superfluid Reynolds number, here we show evidence for a superfluid analogue to the classical dissipation anomaly.
Our numerics indeed show that as the superfluid Reynolds number increases, smaller and smaller structures are generated on the quantized vortex lines on which the superfluid vorticity is confined, balancing the effect of weaker and weaker dissipation.
22 febbraio - Angela Monti, IAC-Bari
Model Order Reduction for Turing pattern approximation in reaction-diffusion PDE systems
Abstract
We investigate a suitable application of Model Order Reduction (MOR) techniques for the numerical approximation of Turing patterns, that are stationary solutions of reaction-diffusion PDE (RD-PDE) systems. We will consider in particular, the Proper Orthogonal Decomposition (POD) and the Dynamic Mode Decomposition (DMD). Both techniques present inaccurate approximations, therefore we will introduce two novel algorithms that aim at stabilizing the studied problem. In the first part of the talk we focus on the stabilization of the POD-DEIM technique. We show that solutions of surrogate models built by classical POD-DEIM exhibit an unstable error behaviour over the dimension of the reduced space. To overcome this drawback, we add a correction term that provides missing information to the reduced model and we apply the POD-DEIM technique to the corrected model. To further improve the computational efficiency, we propose an adaptive version of this algorithm in time that accounts for the peculiar dynamics of the RD-PDE in presence of Turing instability. We show the effectiveness of the proposed methods in terms of accuracy and computational cost for a selection of RD-PDE systems, i.e. FitzHugh-Nagumo, Schnakenberg and the morphochemical DIB models, with increasing degree of nonlinearity and more structured patterns. In the second part we show some preliminary results regarding a new adaptive algorithm based on Dynamic Mode Decomposition (DMD). DMD is a data-diven technique that allows to find the best linear fit for a given dataset. We propose to modify the method by splitting the time interval into several subintervals to keep a certain level of accuracy. Numerical results will show the efficiency of the shown method. This is a joint work with Alessandro Alla (Università Ca’ Foscari, Venezia) and Ivonne Sgura (Università del Salento, Lecce).
15 marzo - Ludmil Zikatanov, Mathematics Department, The Pennsylvania State University, USA
Titolo: Multilevel methods for nearly-singular problems in mixed dimensions
Abstract
We consider nearly singular problems, that is, problems with operators that are small, but nonsingular perturbations of singular operators. Discretizations of such problems lead to matrices with condition numbers of the system growing rapidly with mesh size and model parameters. This results in slow convergence even when using preconditioners which are optimal when the nonsingular perturbation dominates.
To design efficient preconditioners we follow the theory of the method of subspace corrections and construct block Schwarz smoothers for the underlying multilevel solution method. The blocks are chosen specifically to cover the supports of the vectors/functions spanning the kernel of the singular part of the operator. We demonstrate key features of such solvers on a mixed-dimensional model of electrodiffusion in brain tissue. This is a joint work with Ana Budisa, Miroslav Kuchta, Kent-Andre Mardal (from University of Oslo and Simula) and James Adler and Xiaozhe Hu (Tufts University).
3 maggio - Cristiano Tamborrino, Università degli Studi di Bari
Abstract
Splitting schemes are numerical integrators for Hamiltonian problems that may advantageously replace the Stormer Verlet method within Hamiltonian Monte Carlo (HMC) methodology. However, HMC performance is very sensitive to the step size parameter; in this paper we propose a new method in the one-parameter family of second-order of splitting procedures that uses a well-fitting parameter that nullifies the expectation of the energy error for univariate and multivariate Gaussian distributions, taken as a problem-guide for more realistic situations; we also provide a new algorithm that through an adaptive choice of the parameter and the step-size ensures high sampling performance of HMC. The effectiveness of the proposed is firstly tested on some benchmarks examples taken from literature. Then, we conduct experiments by considering as target distribution, the Log-Gaussian Cox process and Bayesian Logistic Regression.
10 maggio - Hsin-Hsiung Bill Huang, University of Central Florida
Titolo: Mixed-type Multivariate Bayesian Sparse Variable Selection and Spatiotemporal Models
Abstract
We introduce a Bayesian framework for mixed-type multivariate regression using shrinkage priors. Our method enables joint analysis of mixed continuous and discrete outcomes and facilitates variable selection when the number of covariates $p$ can be much larger than sample size $n$. Our model can be implemented using a Gibbs sampling algorithm where all of the conditional distributions are tractable. Theoretically, we show that the posterior contracts around the true parameter in mixed-response models when $p$ grows subexponentially with $n$. To cope with the high computational cost when $p$ is large, we introduce a simple two-step variable selection approach. We prove that our two-step Markov chain Monte Carlo algorithm possesses the sure screening property and achieves a faster mixing time than the conventional one-step Gibbs sampler. Moreover, our two-step estimator can provably achieve posterior consistency even when $p$ grows exponentially in $n$, thus overcoming a limitation of the one-step estimator. Numerical studies and analyses of real datasets demonstrate the ability of our joint modeling approach to improve predictive accuracy and identify significant variables in multivariate mixed response models. Besides, we also develop a Bayesian zero-inflated negative binomial regression model using Gaussian processes for the spatial and temporal random effects. The proposed model improves the model fitting and predictability compared with the smoothing splines random effects by the real data examples including the Florida COVID-19 mortality data and the National Ecological Observatory Network (NEON) biodiversity data.
24 maggio - Francois Baccelli, INRIA- Paris e University of Texas
Titolo: High dimensional stochastic geometry in the Shannon regime
Abstract
This talk will focus on Euclidean stochastic geometry in the Shannon regime. In this regime, the dimension n of space tends to infinity, point processes have intensities which are exponential functions of n, and the random compact sets have diameters of order square root of n. Three basic stochastic geometry models based on Poisson processes will be considered: the Boolean model, the Poisson-Voronoi tessellation and the Poisson hyperplane tessellation.
We will show how to calculate the asymptotic behaviour of the classical quantities of stochastic geometry for these three models in the Shannon regime: for the Boolean model, the volume fraction, the percolation threshold or the size of the connected components; for tessellations, statistics of geometric properties of cells (volume, diameter, etc.).
These questions are motivated by problems in information theory, including the calculation of error exponents for channel coding based on random codes and calculating the distortion in the one-bit compression-based source coding. Survey of work in collaboration with Venkat Anantharam (UC Berkeley) and Eliza O'Reilly (Caltech).
31maggio - Thibault Damour, IHES, Institut des Hautes Études Scientifiques
Abstract
This Gravitational wave signals from coalescing binary black holes are detected, and analyzed, by using large banks of template waveforms. The construction of these templates makes an essential use of the analytical knowledge of the motion and radiation of gravitationally interacting binary systems. A new angle of attack on gravitational dynamics consists of considering (classical or quantum) scattering states. Modern amplitude techniques have recently given interesting novel results. These results are reaching a level where subtle conceptual issues arise (quantum-classical transition, radiative effects versus conservative dynamics, massless limit,...).
28 giugno - Pasqua D'Ambra, Dirigente di ricerca CNR IAC
Titolo: Bootstrap Algebraic MultiGrid Methods in Graph Clustering
Abstract
This Graph Laplacian is a popular tool for analyzing graphs, in particular for graph clustering. Eigenvectors of the graph Laplacian are often used to obtain a geometric representation of the graph which generally enhances cluster properties and improves cluster detection. We apply a bootstrap Algebraic MultiGrid (B-AMG) method which constructs a set of vectors associated with the graph Laplacian. These vectors, referred to as algebraically smooth ones with respect to the B-AMG method, span a low-dimensional Euclidean space which we use to represent graphs with possible vertex attributes. We demonstrate the benefits of our approach for effective cluster detection both in synthetic and in realistic graphs. This is a joint work with Panayot S. Vassilevski from Portland State University and Luisa Cutillo from the University of Leeds.